Unleashing Business Potential with Data Annotation Machine Learning: A Comprehensive Guide
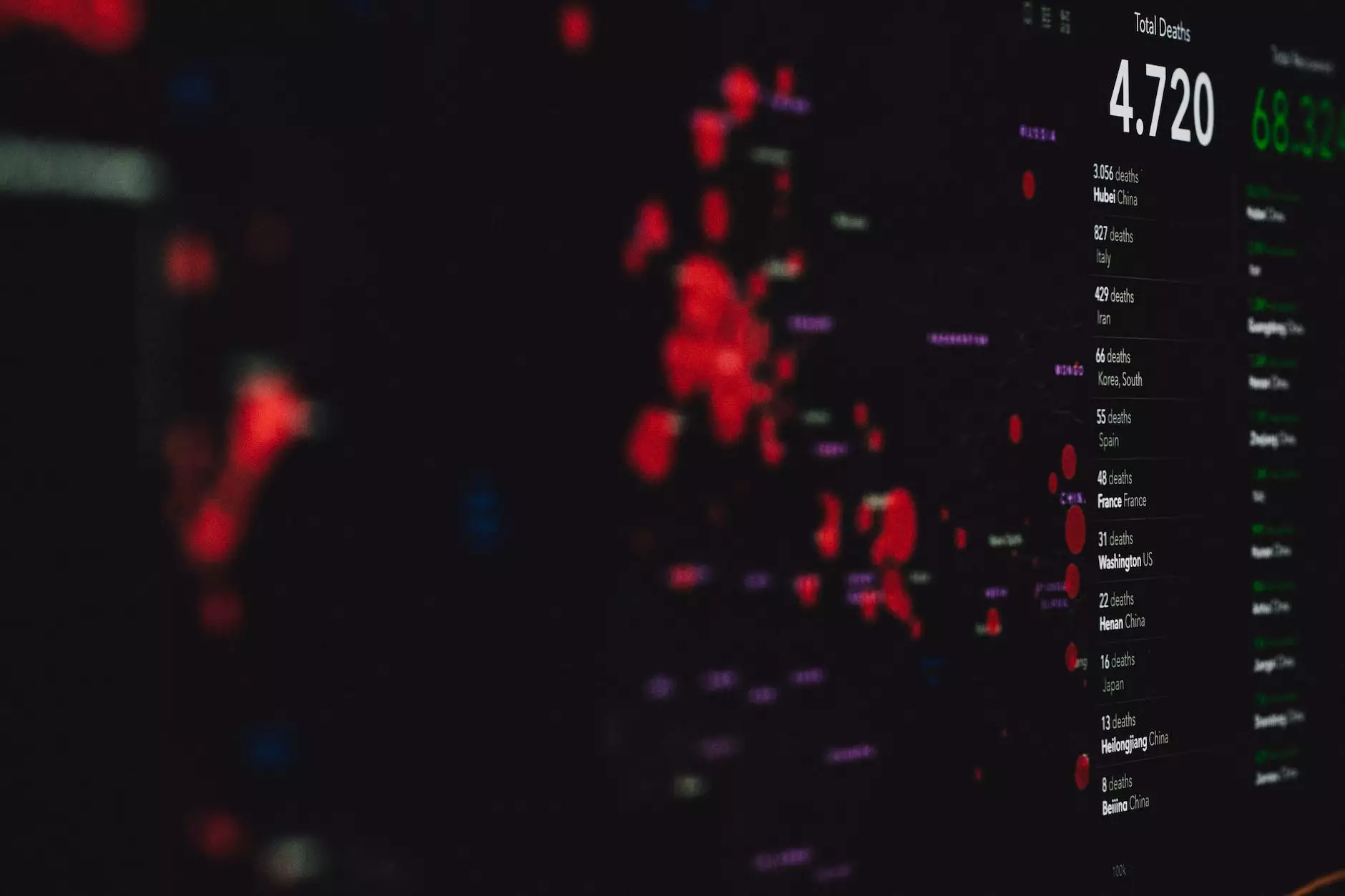
Introduction to Data Annotation Machine Learning: The Future of Business Innovation
In the rapidly evolving digital landscape, data serves as the cornerstone of innovation and competitive advantage. As industries embrace artificial intelligence (AI) and machine learning (ML), the importance of high-quality, accurately annotated data cannot be overstated. Specifically, data annotation machine learning plays a pivotal role in enabling systems to understand, interpret, and act upon complex information.
Businesses across various sectors recognize that effective data annotation accelerates the development of intelligent applications, from autonomous vehicles to personalized marketing. Through meticulous data annotation, companies transform raw data into structured, meaningful insights that power machine learning algorithms, enhance software capabilities, and foster smarter decision-making.
The Critical Role of Data Annotation in Modern Business Applications
Data annotation is the process of labeling and categorizing raw data—images, videos, text, and audio—so that machine learning models can learn from it. As businesses increasingly adopt AI-driven solutions, accurate data annotation becomes the backbone for success in software development.
Why Data Annotation Matters for Business Success
- Enhanced Model Accuracy: Well-annotated data improves the predictive capabilities of AI models, leading to fewer errors and more reliable outputs.
- Reduced Development Time: High-quality annotations streamline the training process, enabling faster deployment of AI systems.
- Competitive Differentiation: Accurate data facilitates the creation of innovative features that set a company apart from competitors.
- Cost Efficiency: Properly annotated data reduces the need for extensive retraining, saving time and resources.
How Data Annotation Fuels Machine Learning in Business Software Development
In the realm of software development, integrating machine learning models rooted in accurate data annotation has become a strategic imperative. Whether developing autonomous systems, natural language processing tools, or recommendation engines, properly annotated data enhances the learning process.
The Impact of Data Annotation on Machine Learning Performance
Machine learning algorithms require massive amounts of data to learn effectively. Data annotation provides this critical labeled data, allowing models to recognize patterns, classify objects, and understand language context. The better the annotation quality, the more proficient the model will be in real-world scenarios.
Key Business Benefits of High-Quality Data Annotation
- Improved Customer Experiences: AI-powered chatbots and recommendation systems depend on annotated data for contextual understanding.
- Automation of Routine Tasks: Object detection and speech recognition driven by annotated data automate repetitive processes, increasing productivity.
- Enhanced Decision-Making: Accurate data enables predictive analytics that inform strategic business choices.
- Innovation Acceleration: With precise data annotation, businesses can develop cutting-edge AI solutions that disrupt markets.
The Process of Data Annotation: From Raw Data to Business Intelligence
Understanding the data annotation pipeline is vital for maximizing ML outcomes. The process involves meticulous steps to prepare data for effective model training:
- Data Collection: Gathering raw data relevant to business objectives—images, videos, text, or audio.
- Data Preprocessing: Cleaning and organizing data to ensure consistency and readiness for annotation.
- Annotation & Labeling: Applying precise labels—bounding boxes, segmentation masks, class labels, transcripts—and ensuring consistency.
- Quality Assurance: Reviewing annotated data for accuracy, completeness, and adherence to standards.
- Model Training & Evaluation: Using annotated data to train machine learning models and assessing their performance for further refinement.
Advanced Techniques in Data Annotation for Business Applications
As demanding AI applications grow more sophisticated, so do the techniques used in data annotation. Here are some state-of-the-art methods that are powering data annotation machine learning today:
- Automated Annotation Tools: Leveraging AI-assisted annotation to speed up labeling processes, reduce human error, and manage large datasets efficiently.
- Active Learning: Using models to identify which data samples need annotation, optimizing resource allocation.
- Crowdsourcing Annotation: Engaging a broad network of annotators for large-scale, diverse data labeling tasks with quality controls.
- Domain-Specific Annotation: Tailoring annotation standards to particular industries, such as medical imaging or autonomous driving.
The Business Advantages of Partnering with Expert Data Annotation Services like KeyMakr
Partnering with an experienced data annotation machine learning service provider offers strategic benefits:
- Expertise & Quality Assurance: Ensuring annotations meet industry standards and are of the highest quality.
- Scalability: Handling large datasets seamlessly as your business grows.
- Cost-Effectiveness: Reducing internal resource burden and avoiding costly mistakes.
- Faster Turnaround: Accelerating project timelines, enabling quicker deployment of AI solutions.
- Customization & Industry Knowledge: Tailoring annotation approaches for specific business needs and verticals.
KeyMakr: Leading the Charge in Data Annotation for Software Development
KeyMakr.com has established itself as a premier provider specializing in data annotation machine learning for diverse industries and applications. Their expertise in high-quality, custom annotation solutions ensures that your AI initiatives are built on a solid foundation.
Why Choose KeyMakr?
- Comprehensive Data Annotation Services: Image, video, text, audio annotations, and more.
- Strict Quality Control: Multi-layered review processes for maximal accuracy.
- Industry-Specific Standards: Medical, automotive, retail, and other vertical expertise.
- Flexible Delivery Models: On-demand, scalable, and tailored to project requirements.
Future Trends in Data Annotation and Business Growth
The field of data annotation machine learning is dynamic, with technological advancements promising even greater business impacts:
- AI-Assisted Annotation Tools: Increased automation in labeling, reducing human effort and error.
- Self-Labeling Data: Emerging techniques enabling models to annotate data autonomously for initial training phases.
- Real-Time Annotation: Fast, real-time data labeling for applications like autonomous driving and live analytics.
- Cross-Industry Standardization: Developing universal annotation schemas to foster interoperability and data sharing.
Conclusion: Embracing Data Annotation to Propel Your Business Forward
In summary, data annotation machine learning is not just a technical necessity but a strategic lever for modern businesses seeking innovation and operational excellence.
By investing in high-quality annotation services, leveraging the latest techniques, and partnering with industry leaders like KeyMakr, your organization can unlock new revenue streams, improve customer engagement, and stay ahead in a competitive market.
In the era of AI-driven software development, meticulously annotated data is your most valuable asset. The future belongs to those who understand that precision in data annotation translates directly into smarter applications, better insights, and ultimately, superior business performance.
Take Action Now
Explore how data annotation machine learning can revolutionize your business software solutions today. Reach out to expert providers, streamline your data pipeline, and position your company at the forefront of technological innovation.